Several concepts from this article
https://www.holisticseo.digital/theoretical-seo/ranking/
are going to be analyzed:
- Reranking methods
- Topical Coverage
- Semantic Content Network
- Historical Data
Suscribe to Topical Authority and Semantic SEO Course
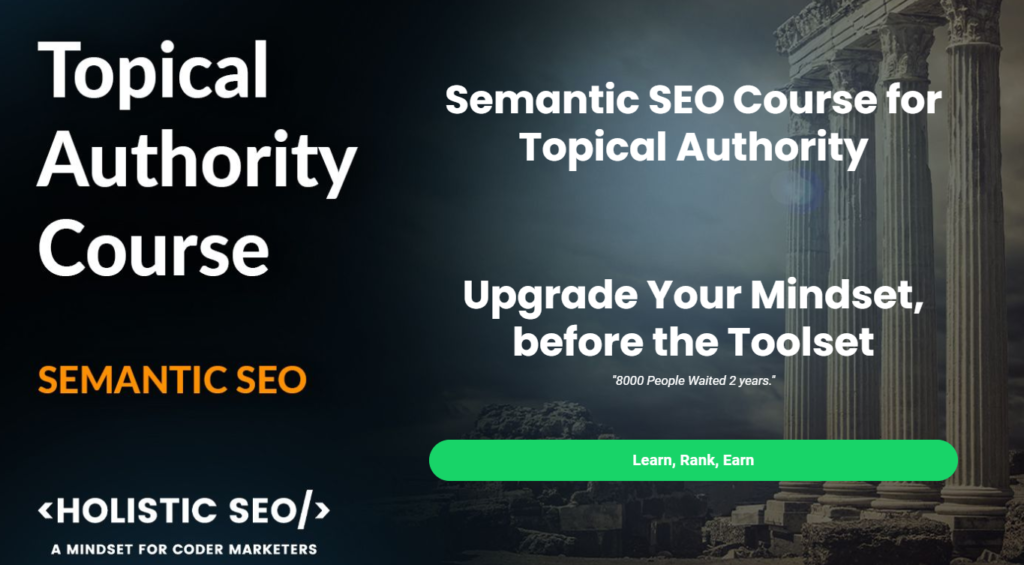
What are the Re-ranking Methods and Criteria of Search Engines?
- Rerank search results by looking at the population or audience segmentation information: Audience Segmentation is the process of creating a demographic profile that includes gender, age, location, interest, income, occupation, character, and condition profile for the larger user segments. The first population assignment can be created with the first ranking score (initial ranking score) during the document retrieval process. When the document is retrieved, it will be matched with an audience, which is called the first population. This matching will generate a “selection score”. The same process will be performed for the second document too. After the second “selection score” is created, the second audience and the first audience will be compared to each other for creating a better-consolidated population and audience segmentation. When the audience profile is finalized, the selection scores for queries and documents will be refreshed, and the reranking process based on audience segmentation will be completed. During the audience segmentation for different documents for reranking by the Search Engine, historical data has been collected and used.
- Reordering based upon implicit feedback from user activities and click-throughs: Google and other search engines can use implicit user feedback and activity on the SERP, and in the web page documents to re-rank the sources on the query results. “Modifying search result ranking based on implicit user feedback and model of presentation bias” patent of Google, and “Query Chains: Learning to Rank from Implicit Feedback” research shows that instead of taking every user feedback into account, taking the implicit user feedback for longer timeline help to decrease the noise while increasing the efficiency. A search engine can understand the typos for queries such as in “Lexis Nexis” and “Lexis Nexus”, or it can use machine learning to evaluate the implicit user feedback. The good part of re-ranking with implicit user feedback for Search Engines is that it decreases the cost of the re-ranking process, and it is document-independent since it only focuses on the users’ behavior instead of the words on the documents. Thus, the relevance algorithms and implicit user-feedback algorithms support and complement each other to improve the efficiency of the re-ranking process.
- Personalized Anchor Text Relevance: Anchor texts can signal the relevance and quality of a web page. In some situations, it can also signal irrelevance or non-quality. Thus, the surrounding text, or the annotation text, and inferred links, mentions, and language understanding are important for anchor texts. When it comes to SEO, there are different types of anchor texts such as exact match anchor texts, generic anchor texts, descriptive anchor texts, and more. And, in the context of the re-ranking process, semantic annotations, and semantic labels can be used to understand the anchor text of a document. Based on personalization, a search engine can weigh some anchor texts more than others, and it can re-rank the search engine result pages’ documents. These re-ranking processes can affect only the users that are the SERP personalized. A search engine can understand a web search engine user’s interest area by checking it past queries, consistent queries, address bar autocomplete options, and bookmarked URLs, or the user can explicitly state it to the search engine. In this context, anchor texts can carry different weights for re-ranking. If this is a general search behavior, the query logs, and the query processing can turn the individual SERP instances into the general samples of re-rankings.
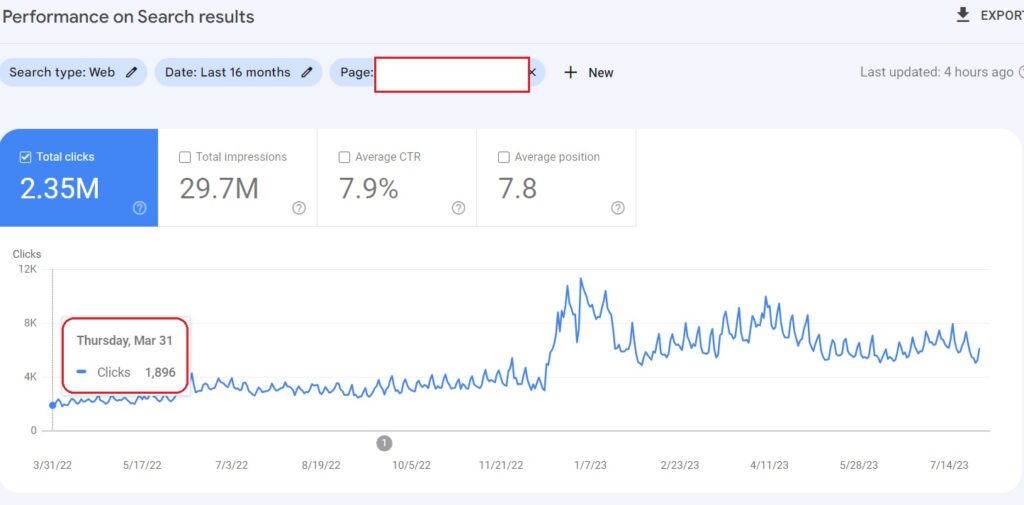
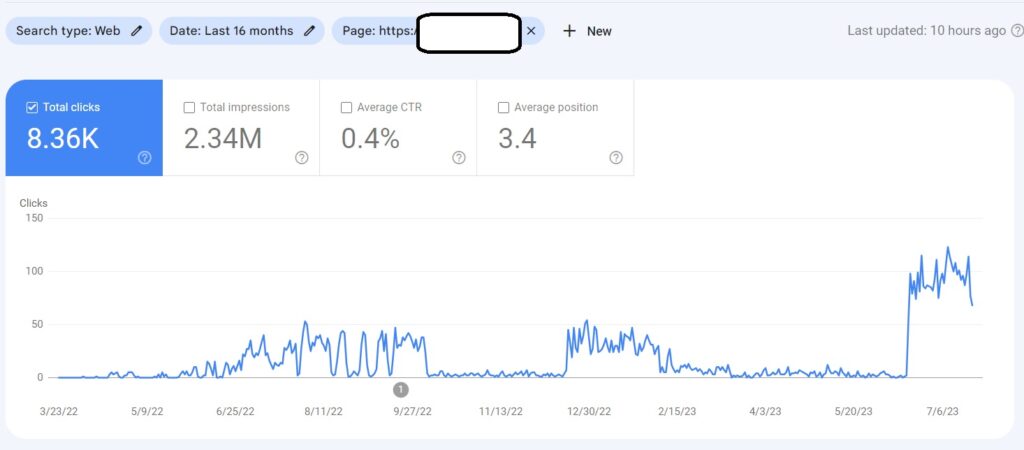
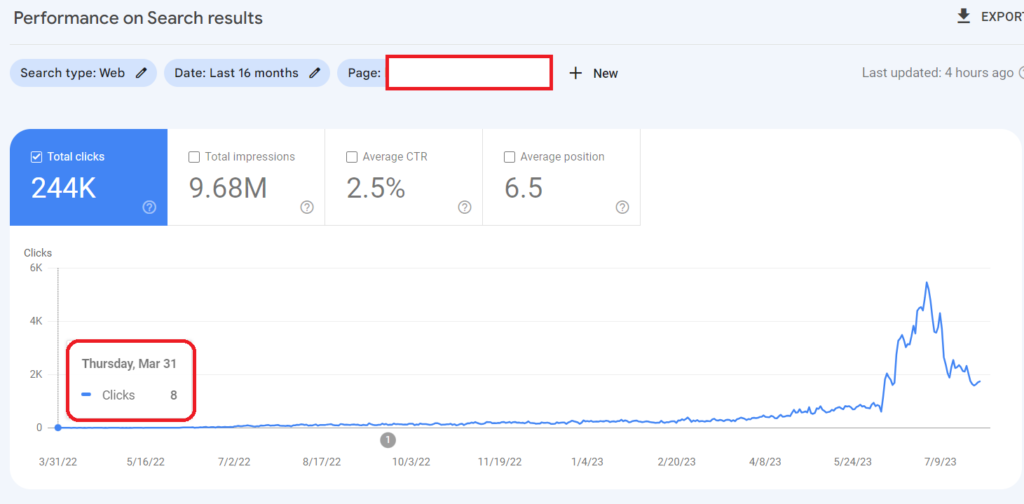
Topical Coverage
Topical Coverage is the definition of how well a source covers a topic well. The informational value of a source for the different sections of a topic will represent its topical coverage. Query Templates, Document Templates, Indexability, and Indexing Cost of the source will affect the topical coverage of the source. In this context, the initial ranking will be influenced based on the query type, document type, and template, along with their relation to each other.
To explain the relationship between initial ranking and topical coverage, you can check the example below.
- A source can initially be ranked for the topic of “ number + pet type + term” between the 1st and the 5th ranks.
- If the query is from a template such as “50 pet type term ( X )”, and if the X is related to other terms from the same topic, a search engine can improve the initial ranking of a document from a source.
- If the query is not from a template, and it is not related to “number + pet type + term”, the search engine can prefer using a lower initial ranking.
- If the source covers related topics by improving its topical coverage, the “non-related query” can have a better contextual relevance for the source, and the initial ranking value can be increased.
A context can be chosen based on the central entity within a cluster of topics, or main entity within only one document, or attribute of the entity that is being processed, or the question and answer format, along with the web page layout.
A context can make topical coverage bigger or smaller. ( Male, female pet )
A source can cover a topic comprehensively based on a context, but another context can improve its topical coverage’s value. An e-commerce site can cover books generally, and comprehensively, but it might not cover the content of the books, reviews of books, authors of those books, or their background information. Thus, topical coverage can not be evaluated without context determination.
Customization based upon previous related queries:
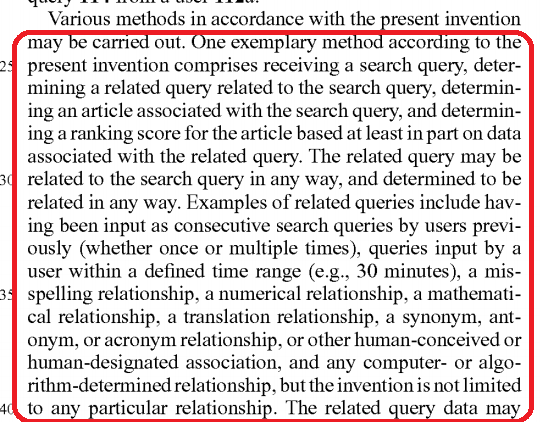
What is a Semantic Content Network?
A Semantic SEO Content Network is a structured content network that describes interconnected concepts, their properties, and relationships, with the possibility of optimal contextual vector, hierarchy, and accurate fact extraction. The Semantic Content Network operates based on the concepts of topical coverage, contextual coverage, and historical data. Topical Coverage is well-known thanks to Topical Authority SEO Case Study, but Topical Coverage can explain the connection between a knowledge domain and the contextual domain along with the contextual layers.
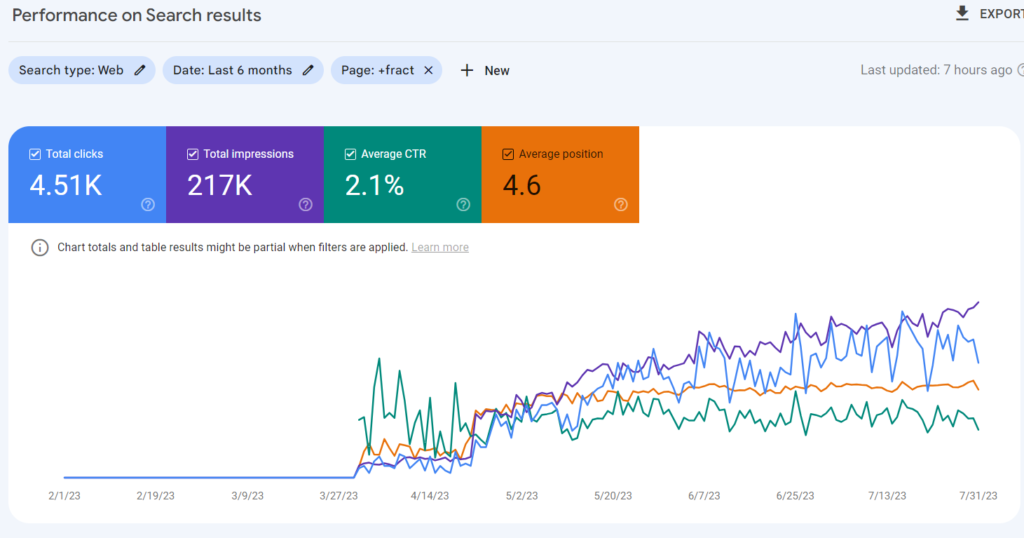
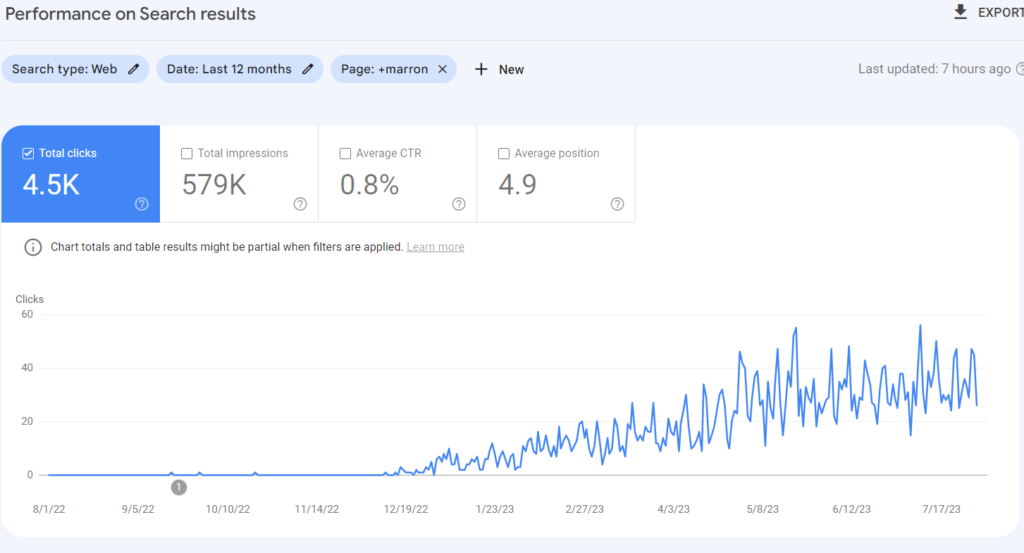
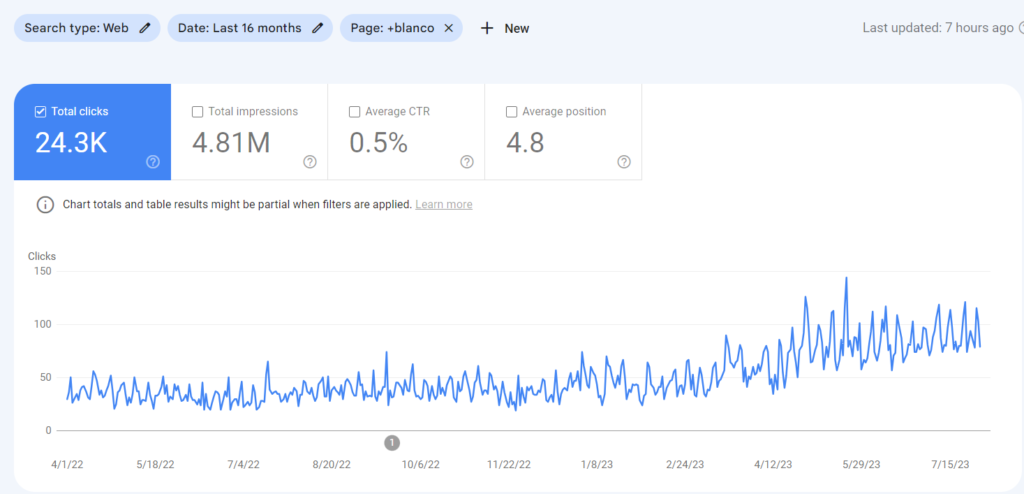
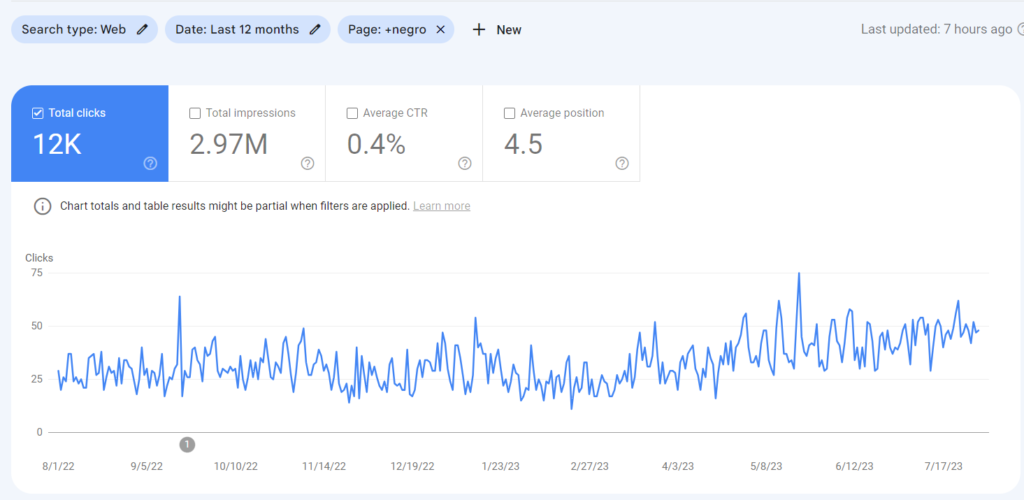
What is Historical Data for SEO?
Historical Data is the second important component for Topical Authority, after Topical Coverage. Historical data can be accumulated with search engine users’ behaviors and their search activity on the SERP. A search engine can gather implicit user feedback from the search engine users based on context, date, and patterns to evaluate the quality and necessity of a document on the SERP. In the context of initial ranking and re-ranking, historical data can affect a search engine’s decision for continuity of the ranking, and average initial and re-ranking processes.
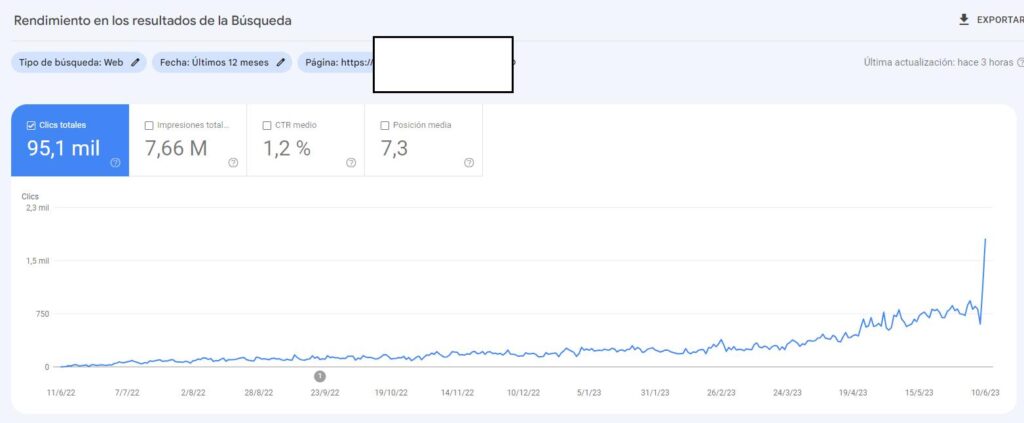
- Historical data is directly proportional to the topical coverage of the relevant website. Having more impressions and SERP Activity values from the same subject will enable the same source to have more historical data. Therefore, sources that cover the same concepts, interests, and question forms better increase re-ranking and initial ranking
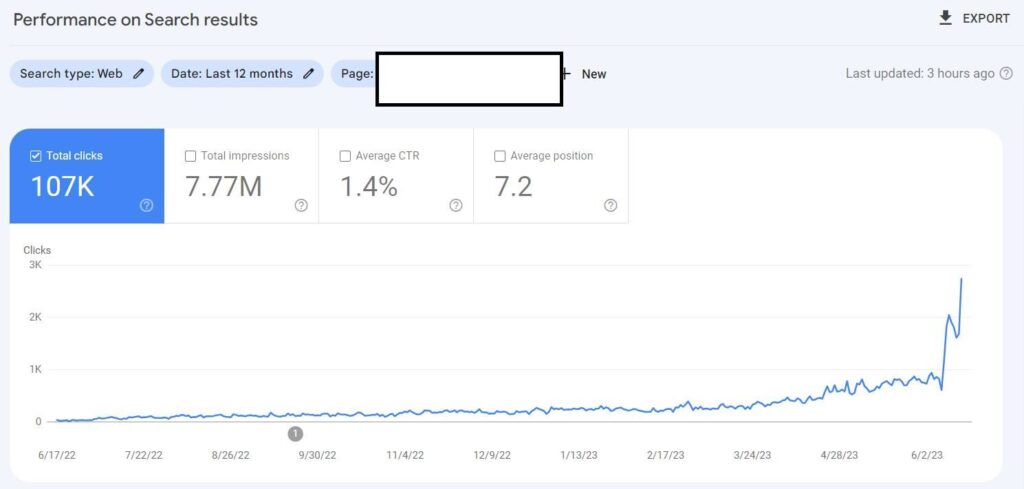
Why is Historical Data Important for a Search Engine?
Historical Data is the most important data for a search engine. With historical data, a search engine can understand what users are searching for, why they are searching, how many ways they are searching, when they are searching, who are searching, with which device they are searching, in what mood they are searching, and for how long they are searching. A search engine’s algorithm is fed with historical data, developed, and improves SERP quality with announced or unannounced updates.
How a Website Can Turn Historical Data and Authority Deficiency into an Advantage for SEO?
A website without historical data might have the advantage of a “low being tested threshold”. “Testing threshold” is the threshold for historical data that determines when a new source (website) will be tested for featured snippets, people also ask questions, and overall better rankings to see whether the source is able to satisfy the users or not. A search engine with probabilistic ranking and degraded relevance score calculation won’t let a source take all rankings over one day, it will happen after a consistent amount of time with a consistent amount of signals. If a source doesn’t have any historical data, it means that the source doesn’t have any negative feedback from the SERP, or quality algorithms of search engines.
In this context, a new source can create enormous amounts of positive historical data within a short time. And, if a source has a new content network with better accuracy, clarity, and informational value for its content, a search engine can decide to try the new source on the SERP against the existing sources. Fastly growing-historical data for a source can trigger a rapid re-ranking process with the help of better initial rankings.
Content structure and strategy
The cost of retrieval refers to the cost that happens during the crawling and indexing process.
The cost of retrieval includes the cost of crawling, rendering, evaluating, associating, indexing, and serving a document for providing value to the users.
In this context, the quality of the document in terms of content, and amount of cost balance each other.
PageRank, or the quality of the information and engagement on the web page, change the cost of retrieval positively.
Only 264 words in the article.
—
Focus on Microsemantics.
Microsemantics will be the name of the next game.
Reason: The bloating on the web will create bigger web document clusters, and being a representative source will be more important.
Thus, micro-differences inside the content will create higher unique value.
Source: https://medium.com/@ktgubur/40-deep-seo-insights-for-2023-482b9bce2b67
—
Competitors | Content strategy | |
Images | Several or dozens | Just 1 image |
Video | No | Yes |
H2 Headings | Several or dozens | 2 Headings H2 |
Table | None | 1 Table |
Structured data | No JSON-LD | JSON-LD |
Words | Thousands | two hundred + |
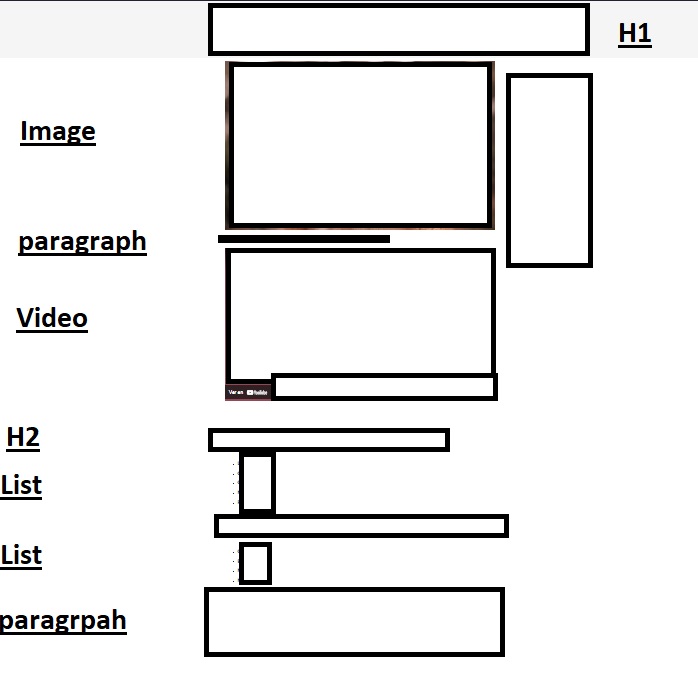
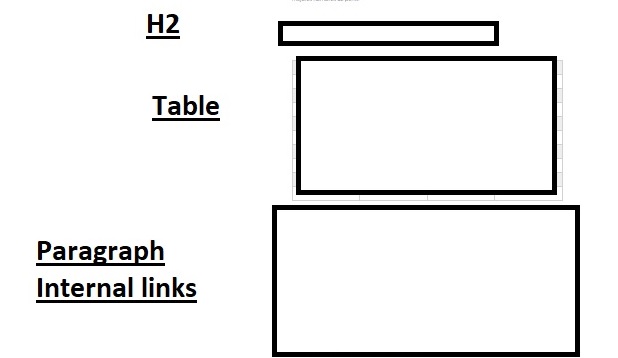
Organic Traffic increase
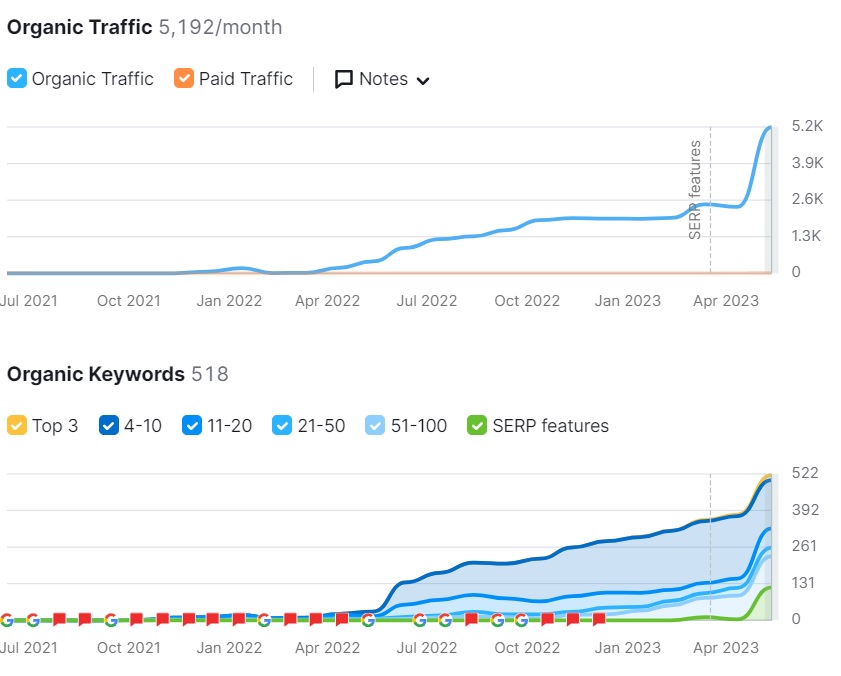
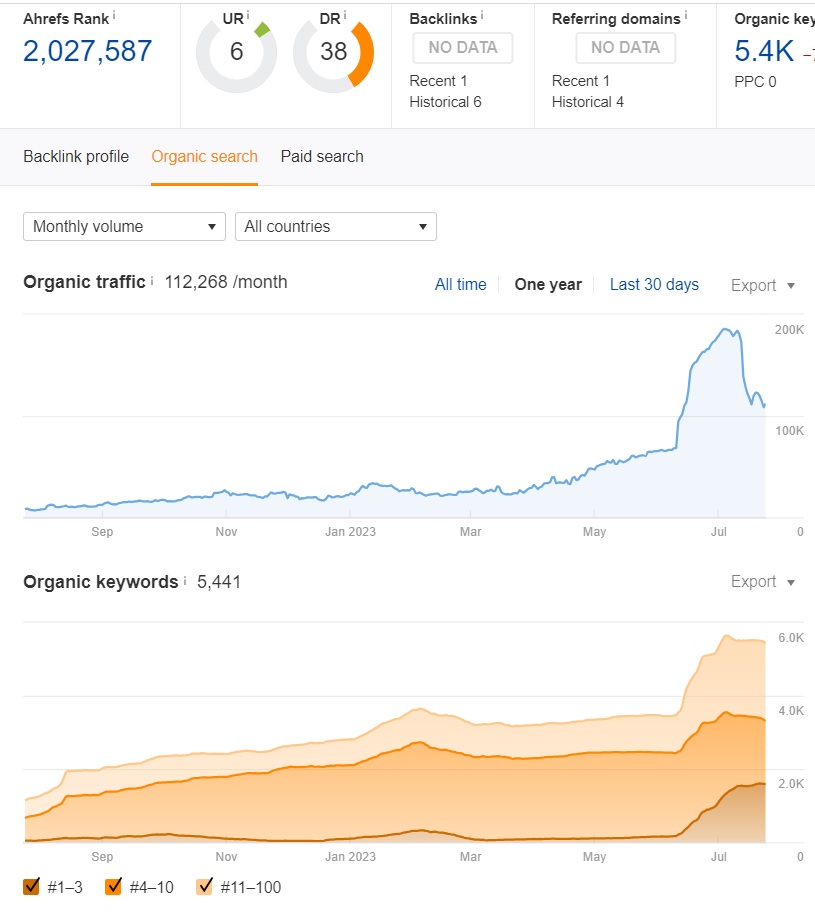
Query Augmentation
Ranking Factor
Put the number in the Title Tag and Heading H1
Combine this with numbers in the content, if it makes sense.
Launch this regex in Search COnsole in order to identify keywords with numbers : [1-9][0-9]*
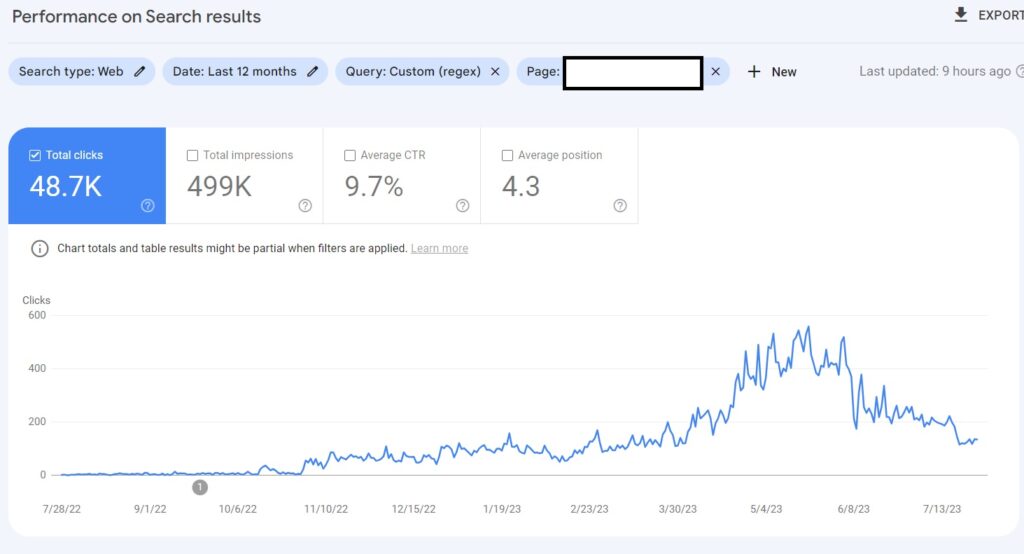
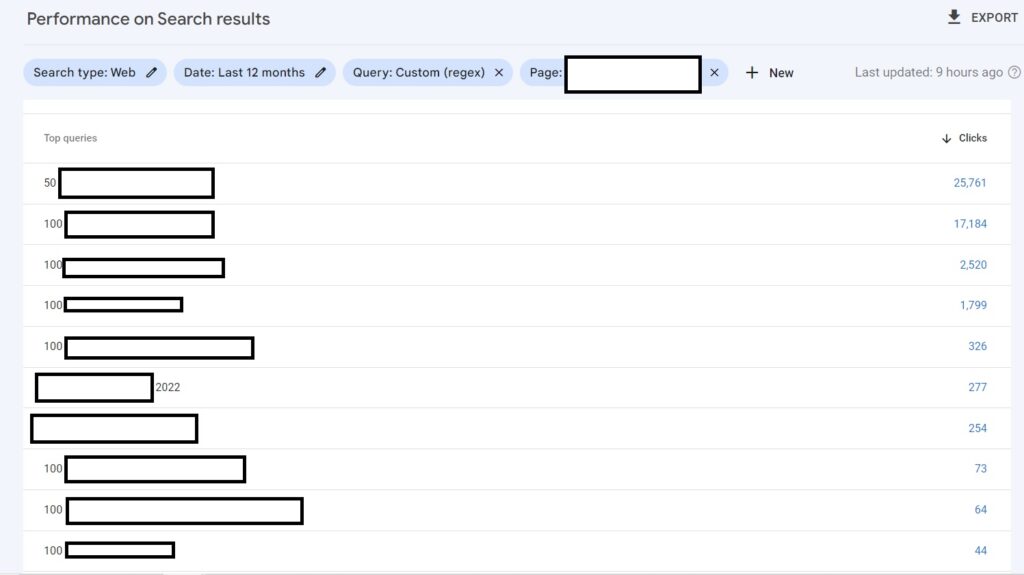
Featured Snippets
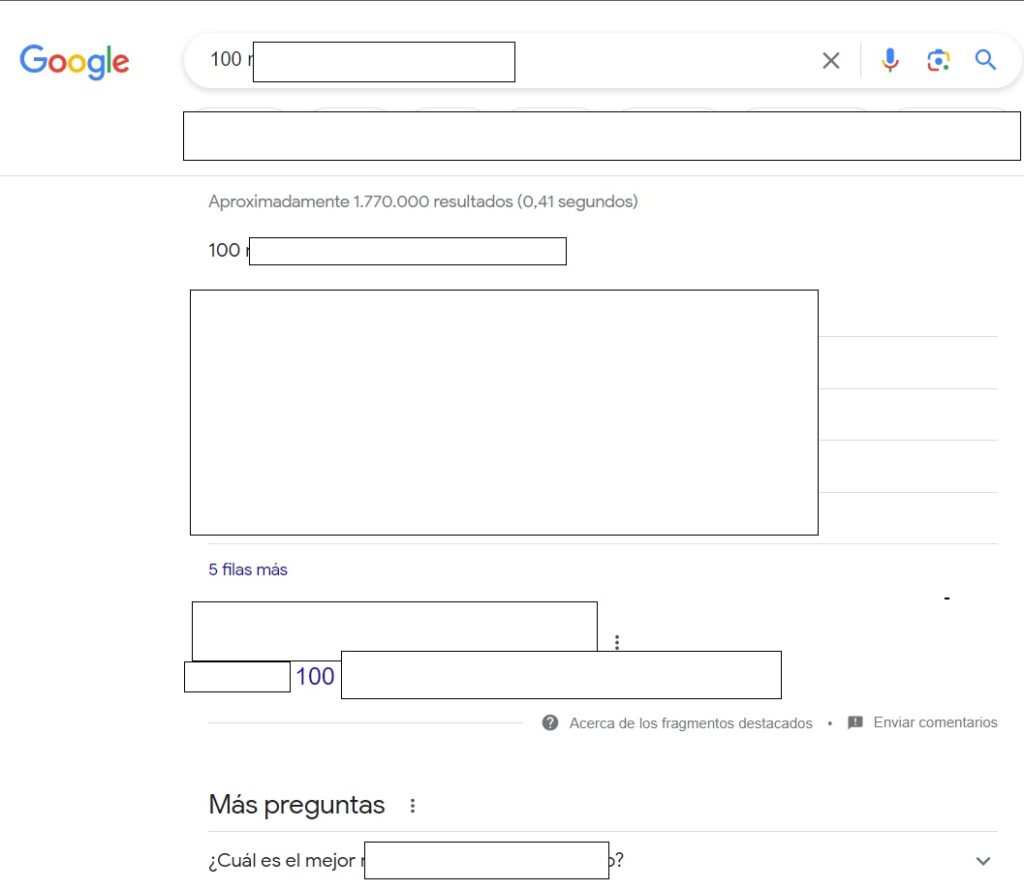
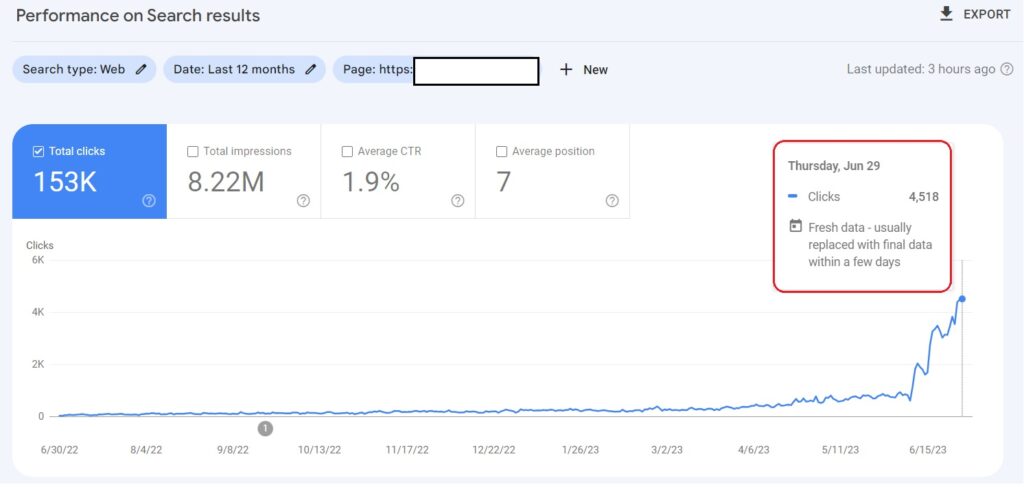
Satisfy User Intent
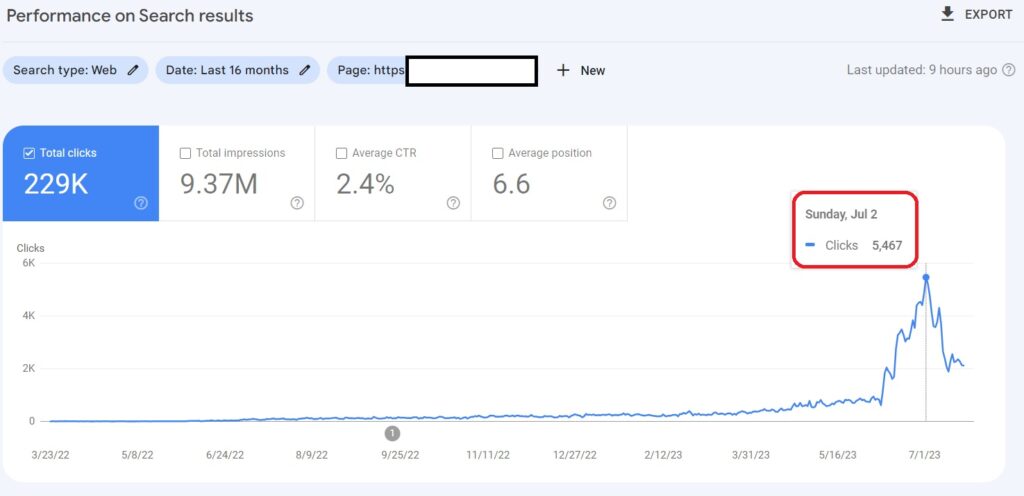
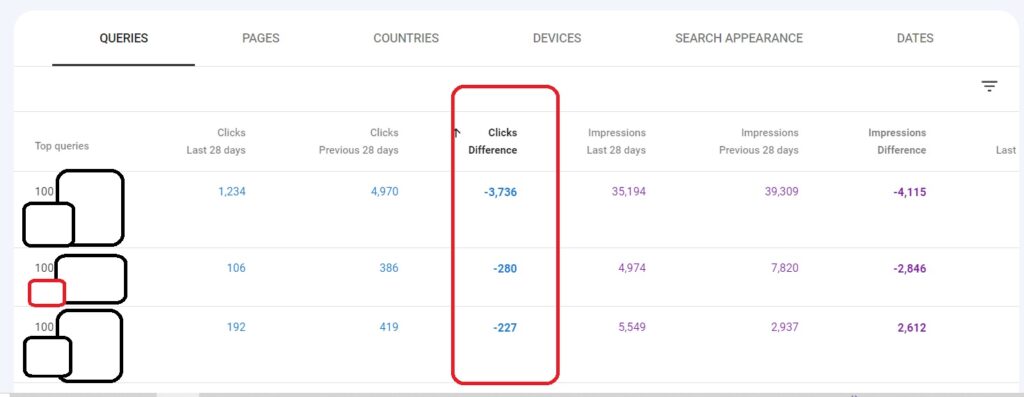
How is it going in Organic Traffic terms?
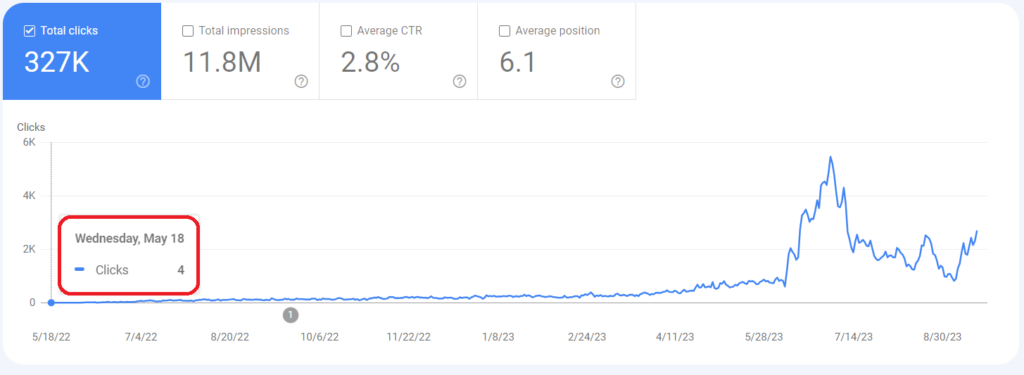
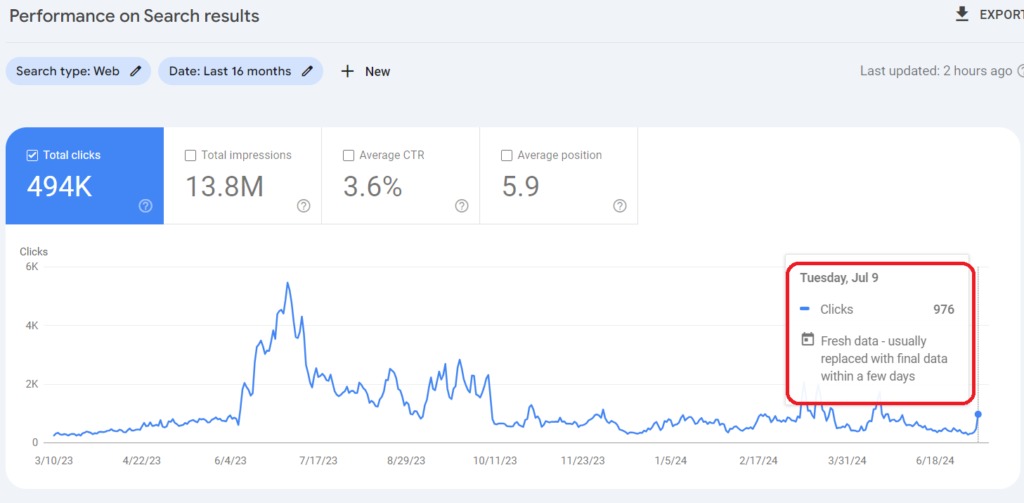
Structured data – (JSON-LD) as a differentiation factor
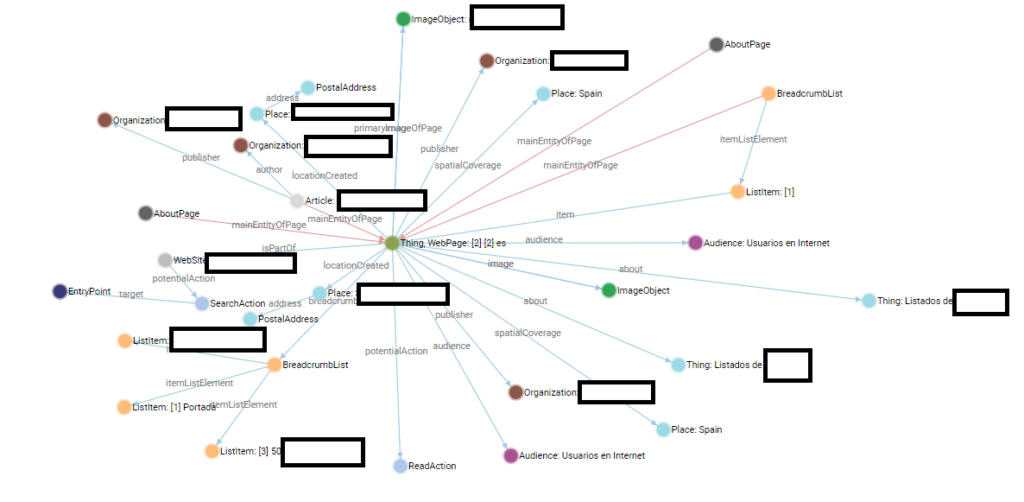
Listen to Pavel Klimakov testimonial